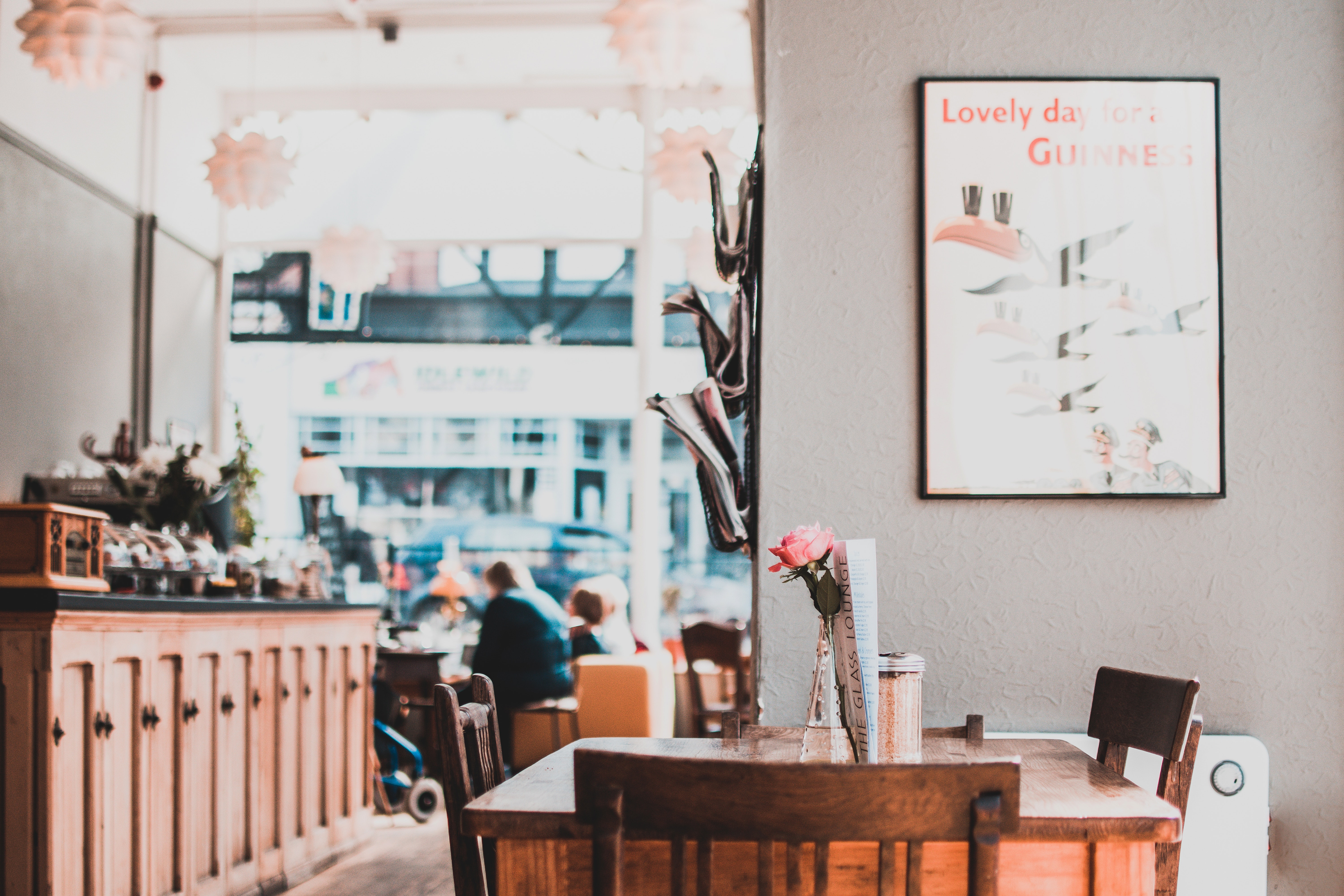
Pure Mathematics
Society wants to know the answer to the most fundamental questions about our Universe; this includes finding the answers to the most fundamental problems in pure mathematics. Machine learning may open the door to this future.
schedule chatMachine learning may facilitate the future of math
Some of the hardest problems in mathematics may be solved using hybrid approaches that involve machine learning.
Generally, this may involve learning implicit understandings that entail knowledge within the domain of a key challenge problem that can be explored subsequently.
Langlands Program
Learning to perform mappings that correspond to relations of the Program may provide a knowledge structure to explore subsequently to further avail.
Hodge Conjecture
Relationships between complex projective manifolds and subvarieties can be explored by generating examples and machine learning sub-problems, from simple algebraic prediction to more complicated mapping between cohomology and Hodge classes.
Riemann Hypothesis
Learning representations and transformations of functions across the class to which the zeta function belongs may permit subsequent inferences (e.g. via transfer learning) specifically about the zeta function.
Advanced toolkit for the future of pure mathematics
Our toolkit can be tailored to suit your specific needs.
Example generation
Work with us to generate training examples using custom or field-standard software for representing objects and attributes.
Attribute learning
Where object attributes can be known in advance, such as Hodge numbers, models can learn about structures by learning to predict these attributes.
Similarity spaces and anomalies
The representations learned of mathematical objects can be taken as points on a manifold that can be considered an organizing structure of those objects, whether in terms of single-query similarity, anomalies, broad groupings, and so on.
Transfer learning
Have a problem for which there are very few examples or very little data? Models previously trained on other problems can subsequently be trained on limited data problems.
Rapid prototyping
We have the tools and experience to prototype innovative methods quickly, giving you answers about usefulness before committing.
Schedule a chat to discuss >>Accelerated Implementations
Our development process supports rapid translation of prototype methods into (sometimes vastly) accelerated forms using GPUs and FPGAs.
Schedule a chat to discuss >>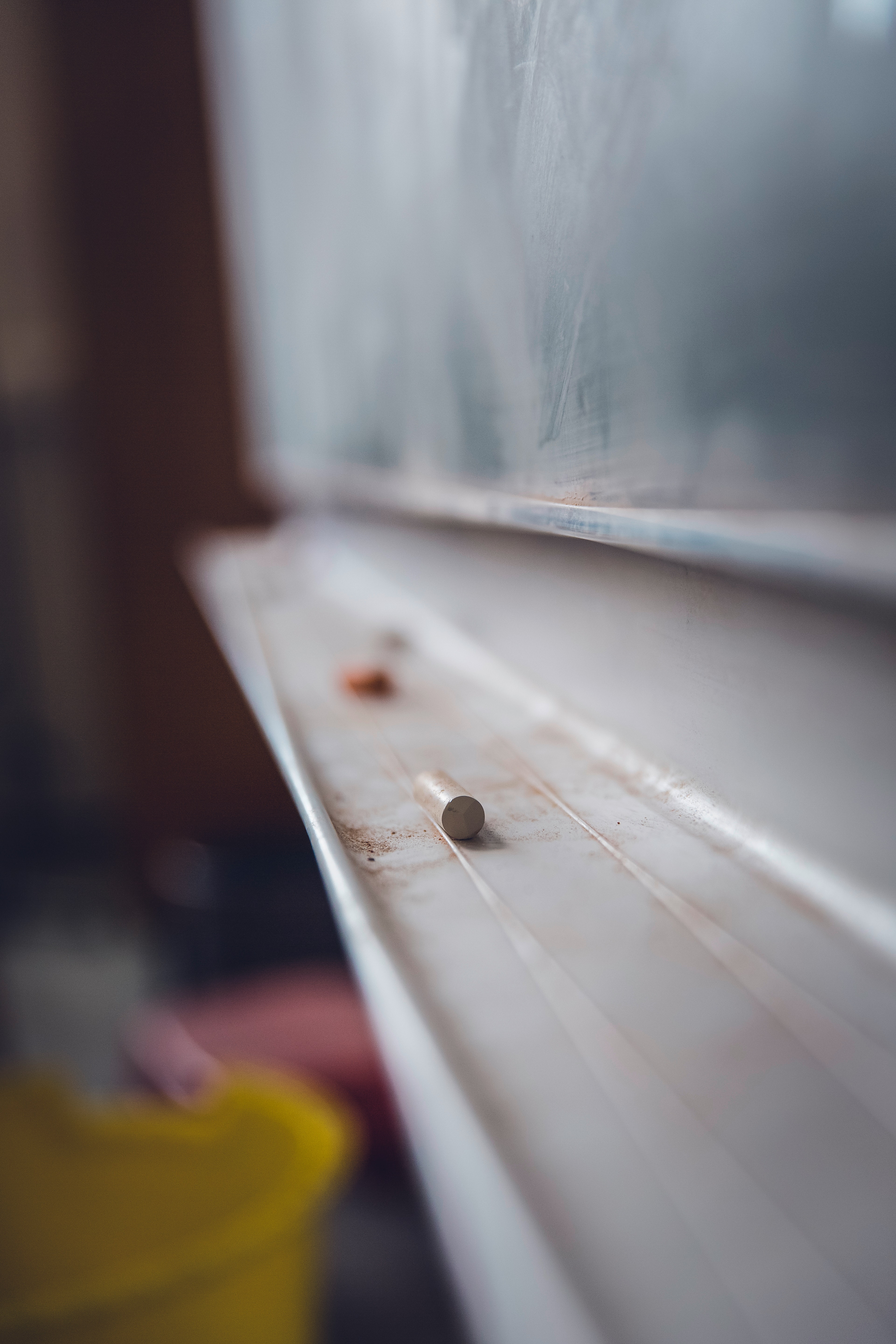
Explore exponentially more approaches
With modern machine learning tools, approaches may be able to be pre-validated or expanded upon before working them through on the board or on paper, giving you far more leverage to try out ideas - experimenting faster and more flexibly. Let's see what we can create together.