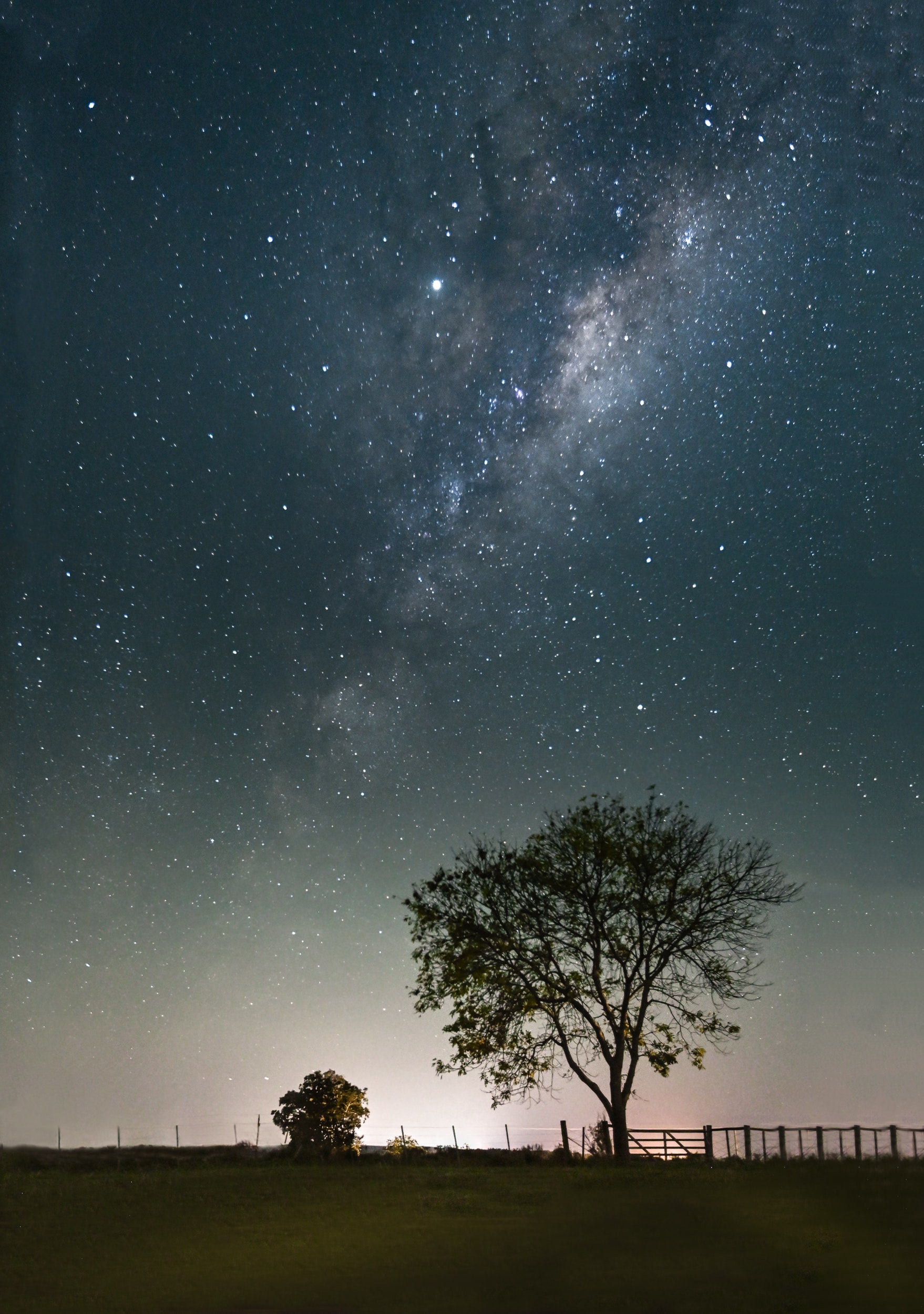
Learn domain objects, then query
Just as with a human learner, models can start by learning about the fundamentals of a domain on a problem well suited to developing such understanding. Thereafter, these can either be explored directly on account of e.g. revealing the structure of sets of domain objects and/or applied to subsequent tasks.
Build domain model
Every domain in theoretical physics involves mathematical objects (e.g. manifolds) and usually operators (fields) on these. Machine learning can be used to obtain fundamental understanding of such manifolds, fields, operations on these, and so on - such as those pertaining to a particular research question. For example, a measure of entanglement entropy.
Explore domain model representations
Once a domain model has been learned, one can explore the structure it reveals by interactively exploring the representation space in which the learned representation reside.
Apply domain model
E.g. Perform Monte Carlo roll-outs to obtain distribution of outcomes for a given input.
E.g. Use domain model in transfer learning, i.e. as the basis for learning on a subsequent problem involving the same model.
Schedule a chat with us to talk about how this learning paradigm can be tailored to your problem in theoretical physics.
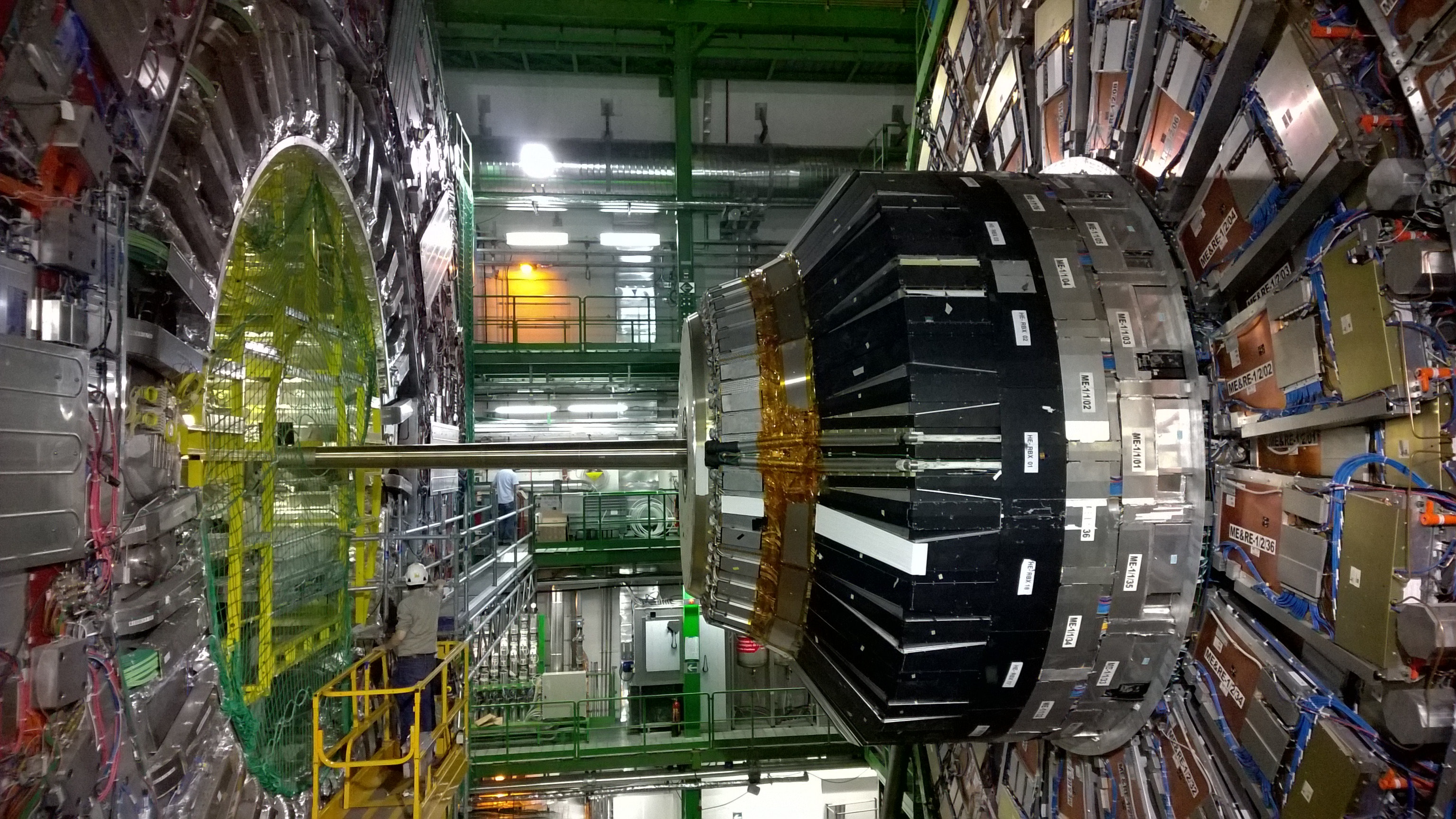
Design and Pilot Project
Following a collection of specs and definitions of goals and metrics we will perform a usefulness and feasibility pilot study to determine early whether to conduct the full project.
Full-scale project
A full-scale project will typically involve progressive one-month iterations of model training and evaluation within the scope of three month (quarter) goals. Iterations particulars are project dependent.
Publication
Complete the project by submitting a publication to a high-impact journal that was identified at the start of the project as the intended journal of publication. Depend on us for the completion of all relevant portions.
Retrospective / Follow-up
Work with us three months following the completion of the contract to provide information on how you felt the publication and/or project translated into broader benefit to your group.
Emerging research opportunities
It's an exciting time to be in theoretical physics; below are some specific examples of projects that are feasible today.
How many of the manifold or domain properties pertaining to AdS/CFT can be machine learned? These may include topological properties, field transformations, scalar measures, and so on. Having learned these fundamentals of the domain, what subsequent problems can be solved pertaining to AdS/CFT?
Given an existing object, what symmetries or symmetry structure exists within this object? What about one or multiple large categories of object? Are there unusual relationships between the symmetries or symmetry structures of the elements of various object categories?
Particle physics is a classic example of a problem where a massive amount of data can be represented in the search for anomalies that in turn can inform theory improvements. To what extent to existing models concur with the learned distribution of even frequencies or event novelty?
Machine-learn attributes and a measure of similarity of a set of manifolds, such as Calabi-Yau three-folds; explore manifold of learned representations, providing a taxonomy of this class of object.
Machine learning a computational tool emulator for Ricci Flow that can in turn be used to infer Topological Quantum Field Theory re-parameterizations.
Learn an implicit model of fluid dynamics using next-frame prediction given an observed time series; subsequently use this to train a predictor of fluid temperature.
Let us know what matters to you; we can easily generate a menu of a diversity of project opportunities to form the basis for an interesting discussion.
Advanced toolkit for the future of theoretical physics
We have an extensible mathematical and applied machine learning toolkit that can be customized to facilitate advances at the forefront of theoretical physics.
Emulating and improving theory tools
Many existing theory-domain software tools that can perform a task (such as computing Ricci Flow transformations) can be emulated using machine learning, in many cases to perform the same simulation but far more rapidly or perhaps in order to be the basis for subsequent understanding.
Attribute Prediction
Given an object, predict the attributes of that object that are known either through alternative computation or experimentation. Either directly apply the means to predict such an attribute or use this as a pretraining problem.
Anomaly Detection
Machine learn domain models providing representation structure against which new or existing examples can be compared to determine whether they are anomalous; for example, events in massive particle physics experiments.
Understanding time-series dynamics
Where time-series can be collected, whether simulated or experimental, models can learn implicit understandings by generating equivalent sequences. Learned models can then be either explored in terms of representations
Rapid Prototyping
We have the tools and experience to prototype innovative methods quickly, giving you answers about usefulness before committing.
Schedule a chat to discuss >>Accelerated implementations
Our development process supports rapid translation of prototype methods into (sometimes vastly) accelerated forms using GPUs and FPGAs.
Schedule a chat to discuss >>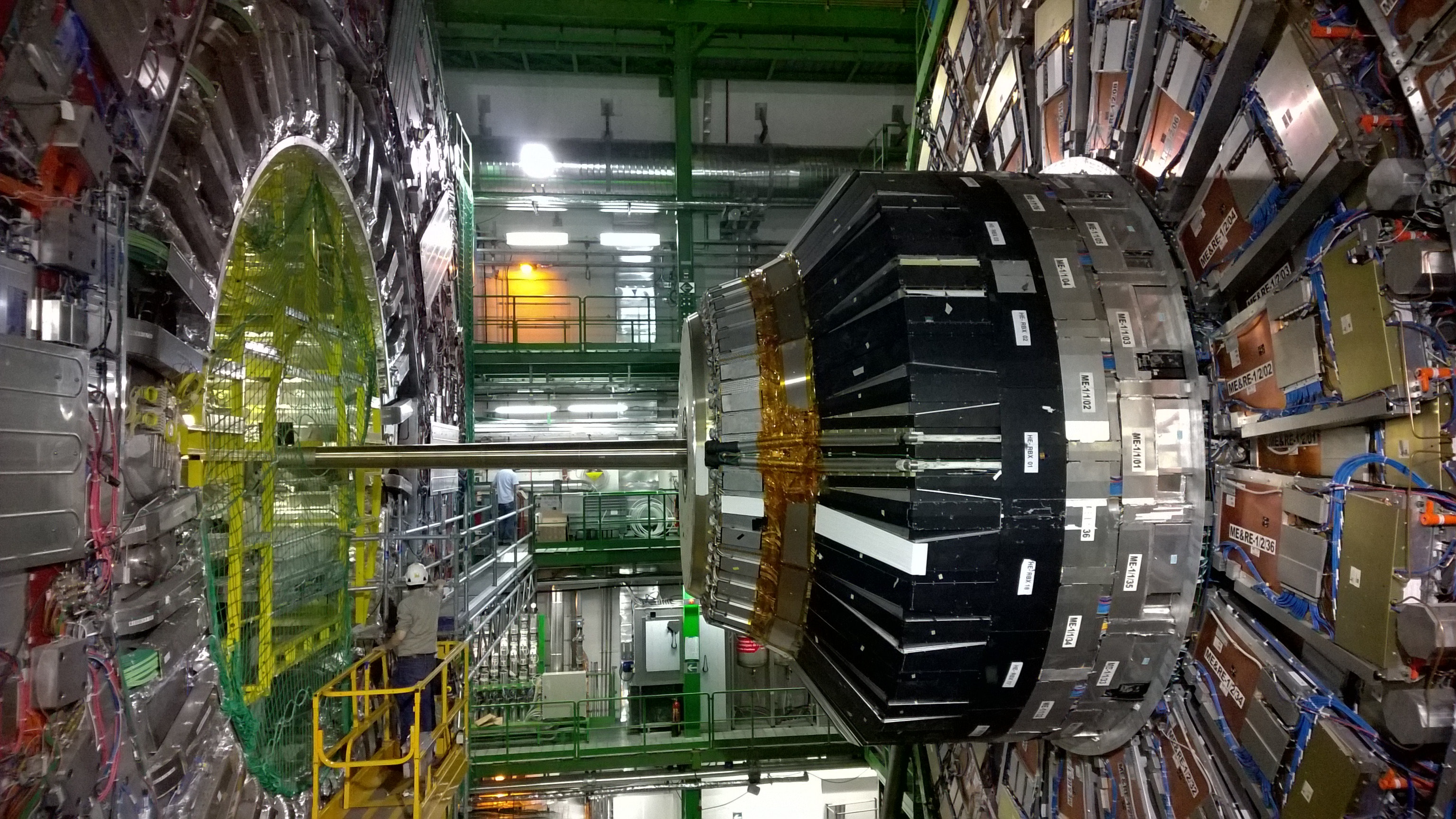
What more can we discover?
More deeply understand the mysteries being uncovered by today's advanced technologies